This post was authored by Brian Courtney, VP and GM at Resideo. He previously was CEO of LifeWhere and served as general manager of industrial data intelligence at GE Automation.
We have come a long way from dumping information into databases never to be seen or heard from again, but we still have a long way to go. Today’s software infrastructures for remote monitoring and diagnostics (RM&D) are moving from analyzing real-time data to mining larger data sets for additional knowledge of equipment. Industrial big data infrastructures are being built for storing and processing extremely large volumes of data sets.
As the need grows for real-time analysis, it will no longer be acceptable for these two infrastructures to be kept in distinct silos. Big data storage and fast processing capabilities will have to be merged into one hybrid system.
Today’s high throughput infrastructures for real-time analysis allow companies to use advanced and predictive analytics to adjust equipment at high speed in a way that a human operator could never achieve. Done with real-time data, industrial process optimization analytics run as closed-loop systems often at the point of control within the controls hardware. To reach this stage of industrial analytics, there are four steps every company must take:
Step 1: Collect the right data
The first step begins with basic monitoring of critical assets to see what happened in the past and in near real time. To accomplish this level of monitoring, you need to instrument your critical equipment with sensors and control networks. Online systems, at the point of control, often include supervisory control and data acquisition systems.
If you are like most industrial businesses, the volume of data you have to manage is ever increasing. To stay competitive, you need to understand and control your operations by efficiently collecting more and more critical data and maximizing its value.
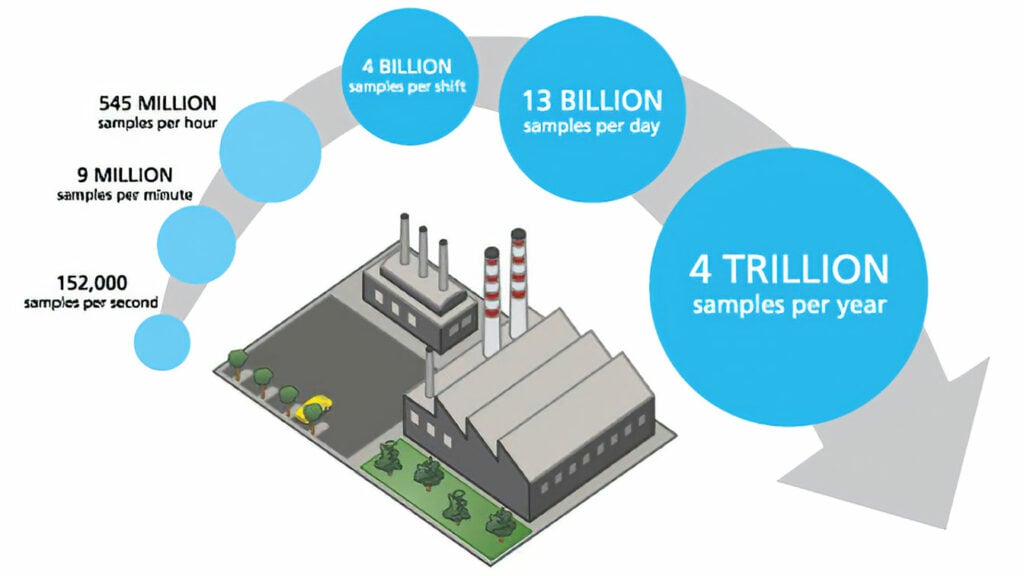
Step 2: Store it properly
So, you have tons of raw data. Where do you go from there? The second step in the journey is to deploy a storage system that can help you effectively leverage raw data from devices such as sensors, meters, and other real-time systems, to improve production. Software solutions are available that easily integrate into any company’s enterprise systems portfolio, offering clear value for logging, storing, and retrieving high volumes of process time series data. Meanwhile, relational databases (RDBs) are designed to manage relationships between contextualized data collected by enterprise historians.
Determining which system works best comes down to what type of information your facility values the most. For example, if you need to make decisions in real time based on time series data, a historian’s capability to analyze high volumes of time series data is right for you. If you need to answer operator queries such as, “What customer had the largest energy demand?” then adding an RDB might be a good solution for your operation. Most software offerings have both a historian and an RDB solution for alarm and event data. It is important to note that you should choose the right data storage for the right type of data: time series into historian, relational into RDB. In fact, some solutions operationally model manufacturing from time series data in a historian married with relational data either automatically or manually entered and then stored in an RDB.

Step 3: Analyze it
Once the data has been stored, the third step is to analyze it. There are many applications for analyzing industrial data, including the performance, quality, and efficiency needs of an operation. For the purposes of an RM&D operation, asset performance is one of the top applications. This starts with equipment reliability, making sure the assets are available when they are supposed to be available, or in simple terms “eliminating unplanned downtime.” The next step can be taken by stabilizing the process itself and reducing variation, which is the primary cause of downtime, among other things like quality issues and energy waste. The final step is to optimize the process running on the asset before getting into area- and fleetwide applications.
Step 4: Get it to the right people at the right time
The data has been collected, stored, and analyzed in a meaningful way; the next and final step is to deliver it to the right person at the right time. This is where people and processes form a collaborative environment between the monitoring and diagnostic (M&D) center and the maintenance crews on the ground. Give foresight, or in plain words, time to the people responsible for maintaining their equipment to analyze the situation. They take the case data from the M&D center and perform insight analysis with visualization tools on high-fidelity data sets to determine if a maintenance action is required. This allows them to schedule during a planned downtime, avoiding expensive trips or unplanned downtime.
By completing the four steps in this road map to value, operators can unlock the value of their data. Empowered by the new data, maintenance staff can identify malfunction causes and use analytics to avoid the problem in the future.
Predictive analytic software can even learn normal equipment behavior, and then predict future behavior. This class of analytic software leverages multivariate analysis techniques, where very complex relationships in data can be identified to predict future states based on any variation in input. For example, ambient temperature can be taken into consideration when looking at equipment temperature to understand if the current equipment temperature accurately reflects modeled temperature based on the current ambient conditions,or if the equipment temperature is unusual and requires examination.
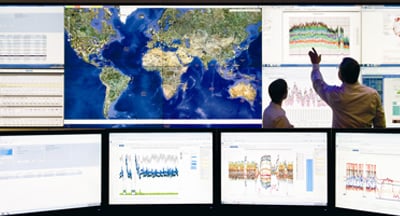
The future of big data analytics (is now)
Over time, industrial data adds up. Depending on the size of your organization, in just a few years, the data sets can become untenable. Enter industrial big data and analytic solutions. The future of industrial big data analytics will focus on hybrid platforms that are a tightly coupled combination of a high-performance operational data management system and cloud-based data storage that leverages technologies (e.g., Hadoop), integrated to achieve the goals of real-time analytic execution on massive data sets. While these components all work in tandem to solve complex challenges, a single interface layer is needed to keep the access simple. Keeping it abstract allows users to insert data, run queries, and invoke analytics without having to understand data formats and the differences in storage mechanisms.
Once the data has aged, such that it is no longer required for near real-time analysis, the data can then be transitioned into a long-term, Hadoop-based storage platform. To extract value from multiple data sources, proper data integration, metadata management, and master data management is key.
Bridging the big data gap
Industrial big data is taking massive strides forward, but the industry is just scratching the surface on its true potential. Today, it is about gathering more datathan you have ever been able to accumulate before and doing it much more quickly. Analysts can compare and correlate years of diverse historical data, creating a myriad of new analysis possibilities. As a result, operators can rapidly detect trends and patterns never before detectable to better understand how equipment and processes are running versus how they should be running and to help prevent maintenance issues before they happen. Tomorrow, all these capabilities will be integrated into one unified platform with combined capabilities. With a unified platform, big data will be put to effective use, and a company will be able to deliver substantial top- and bottom-line benefits.
Some of the key aspects of leveraging big data are to also understand where it can be used, when it can be used, and how it can be used. The value drivers of big data, such as creating strategic value and improving efficiency, should be aligned to a company’s strategic objectives. Strategic value can be created through innovation, acceleration, collaboration, new business models, or new revenue growth opportunities, whereas improving efficiency can be achieved by increasing revenues, lowering costs, increasing productivity, and reducing risk.
About the Author
Brian Courtney is VP and GM at Resideo. He previously was CEO of LifeWhere and also served as general manager of industrial data intelligence at GE Automation. He has more than 20 years of experience working in the software industry. Courtney founded a company in the early 1990s and subsequently consulted for 10 years. He has a B.S. and M.S. in computer science, and an MBA from MIT.
A version of this article also was published at InTech magazine.